Charna Albert
February 2022—In a survey of the international pathology community on the integration of artificial intelligence into diagnostic pathology practice, 80 percent of the 487 respondents predicted integration within the next five or 10 years. Seventy-one percent indicated AI tools could increase their diagnostic efficiency (Sarwar S, et al. NPJ Digit Med. 2019;2:28). In a review of AI in anatomic pathology published last fall, the authors detailed what it will take to get there.
AI use for clinical work, the review authors write, should be “affordable, practical, interoperable, explainable, generalizable, manageable, and reimbursable” (Cheng JY, et al. Am J Pathol. 2021;191[10]:1684–1692). The domain expertise of pathologists is central to design and development. In addition to the needed buy-in and guidelines, they write, caution is needed in implementing machine-based assistance in clinical settings, “as pathologists’ diagnostic decisions are prone to be influenced by AI, introducing novel sources of bias” (Kiani A, et al. NPJ Digit Med. 2020;3:23).
Despite the largely positive attitudes toward AI tools of those responding to the international survey, 48 percent of respondents felt that diagnostic decision-making should remain a predominantly human task. Twenty-five percent said it should be shared equally with an AI algorithm.
Though some pathologists may fear being supplanted by AI, says Liron Pantanowitz, MD, MHA, director of the Division of Anatomic Pathology, University of Michigan Health, and one of the review’s coauthors, “we’re far away from that time. The people making AI algorithms are not making them to replace us. They’re making them to assist us, which is a good thing for now.” Furthermore, he says, most vendors are developing what’s called “narrow AI.”
“Let’s say you have to diagnose prostate cancer. You train a prostate algorithm to look at tissue, find abnormal glands, decide if they’re atypical or not, if they’re atypical how bad, and then you can get the grade.” Such an algorithm is trained to do one task, he says. “And that’s all it will do—very well and reproducibly, but it’s not very broad. If there’s something else in that tissue or biopsy, the algorithm won’t pick it up because it’s not designed to catch everything. It’s not going to have real intelligence.”
Dr. Pantanowitz and coauthors say the ultimate test of an AI-based system is whether it can be integrated into pathologists’ workflow and that computer-assisted automated Pap test screening was an early success story in this regard. Hologic, maker of the ThinPrep imaging system, has now developed a new deep-learning-based and fully digital cytology platform, known as Genius Digital Diagnostics. “We’re testing their product in our lab. We’ve asked for the scanner and AI, and we’re training everyone to do the validation,” Dr. Pantanowitz says of Genius.
Michael Quick, VP of research and development/innovation at Hologic, says Hologic has developed for Genius a scanner that uses volumetric imaging to capture a digital three-dimensional image of the cellular material. “What allows us to do that is capturing the full depth between the top of the glass and the bottom of the coverslip. So you can think of it as a kind of CT scan of a microscope slide.”
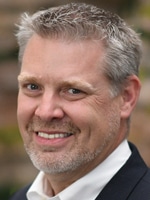
Quick
To make the massive amount of data captured clinically relevant, the scanner then collapses the three-dimensional image into a single two-dimensional representation, with all cells in focus in a single plane. “It allows the user to quickly get a good representation of the cellular content without having to focus up and down on the individual cells,” Quick says. The digital system captures the full cellular detail digitally. “But it’s not just capture it, analyze it, then discard it. We’re retaining it, so now the user can make the diagnosis on a high-resolution monitor.”
The ThinPrep imaging system narrows the cellular content to about 20 percent of the slide for review. “With the Genius platform,” Quick says, “we’re narrowing that even more, with better AI, to get a single gallery of about 30 images of individual cells or cell groups to make the diagnosis.” Genius is CE marked for diagnostic use in Europe, and Hologic is pursuing a regulatory path in the U.S.
Paige received de novo approval from the FDA last September for Paige Prostate. The pivotal study submitted to the FDA found that when pathologists were aided by Paige Prostate, there was a 70 percent reduction in the number of false-negatives, Juan Retamero, MD, Paige’s medical director, says. “This was due to improvements in sensitivity and specificity compared to when pathologists read the same cases without AI assistance,” he says. The study has been submitted for publication.
Developing deep-learning algorithms requires a data labeling step (malignant versus benign, necrosis versus fibrosis, for example), and this process is laborious, Dr. Pantanowitz and coauthors write, “especially considering the large number of images and significant person-hours required for review and annotation.” The annotation process creates a bottleneck and is “almost by definition a limiting process and one of the main problems of supervised learning,” Dr. Retamero said in a presentation at the Digital Pathology Association’s 2021 Pathology Visions conference. Paige didn’t train its algorithm by showing it annotated pixels. Instead, it employed multiple instance learning, a weakly supervised deep-learning approach that uses only the diagnostic report as labels for training.
“What we do is show the whole slide images and corresponding pathology report to the computer and let the computer figure out what’s going on,” Dr. Retamero explained at the conference. “So essentially the model learns from the pathology report.” This means that the model also learns from all the associated processes that may have been reflected in the report, such as additional stains and second opinions. “It’s not that the model learns from the immunohistochemistry images themselves,” he said. “It learns from whatever the pathologist put in the report, which of course may include information from other sources and not just the H&E.”
The Paige Prostate algorithm was trained on 32,300 slides (from 6,700 patients) originating in multiple laboratories. “So the amount of variability we are exposing the model to is incredible, and this is thanks largely to multiple instance training,” Dr. Retamero said. Annotating that number of slides isn’t feasible. With the alternative multiple instance approach, he said, “the system gets exposed to an enormous amount of variability when it comes to patients, preanalytical variables, staining, section thickness. And this amount of variability is the pillar of generalizability”—that is, a model trained on sufficient data “that can be used out of the box in any setting without calibration or further retraining.”
Dr. Retamero likes to think of AI as training a virtual graduate student, “because artificial intelligence isn’t here to replace pathologists,” he said. “It’s here to help pathologists do a better job.” And if a pathologist were to choose a virtual graduate student to screen cases, he said, “which one would be preferred—one who has seen thousands of slides or one who has seen only a few hundred?” That’s the advantage, he said, of the multiple instances trained model.
In the many laboratories that are not fully digitally transformed but have some level of digital operations, AI can be deployed for quality control, Dr. Retamero said. “Labs should strive to achieve the complete digital transformation of their operations, like radiology did decades ago. But for those labs that choose not to do so, artificial intelligence can provide a safe tool to perform quality control of an entire caseload very unobtrusively. This can be done by digitizing the cases and running AI after diagnosis,” he said.
One of the key findings from the Paige study was a small but statistically significant reduction in false-positives when the pathologists were aided by AI, Dr. Retamero said. “This was a pattern that was reproducible across the generalists and the specialists and was present whether they were signing out remotely or on site, regardless of their age and level of experience.” He calls this, along with the “robustness to preanalytical variations,” another important aspect of generalizability, “which is a key benefit of using FDA-approved AI.” They found also that pathologists deferred (to immunostains, levels, second opinions) fewer cases that were clearly malignant and did not warrant deferral in reality, while deferring more cases that would have been wrongly diagnosed as benign.
Dr. Pantanowitz notes it’s unnecessary to wait for FDA approval to begin using AI-based tools, just as it was for digital pathology. For the latter, “Everyone was waiting for FDA-cleared products, and it made things murky and delayed adoption. If an AI product has been verified by the vendor and validated by the lab according to CAP requirements, then you can use it whether it’s FDA cleared or not,” he says.

Dr. Pantanowitz
But validation of AI, he admits, is “another murky area.” Laboratories need to perform clinical validation of AI-based tools on their own data or images. “The problem is there are no guidelines on exactly how to do this.” Dr. Pantanowitz, a member of the CAP’s AI Committee, says he and other committee members have discussed issuing guidelines to lessen confusion, standardize practice, ensure safety and good oversight, and promote adoption. But they decided not to do so at this point, he says, “because there’s no evidence out there to support our recommendations should we develop any, because very few labs are using AI and certainly not publishing about it.” The committee is working on a paper on principles based on good laboratory experience, “but it won’t be a formal guideline.” In the paper, the committee will point to CAP accreditation program checklists that are relevant to AI, for labs that are using AI for a particular task. “But that’s just the existing checklists,” Dr. Pantanowitz says. “There aren’t checklists yet for AI.”
Nor is there reimbursement for AI, though a precedent has been set with machine-learning algorithms to quantify biomarkers such as ER, PR, and HER2 for breast cancer, Dr. Pantanowitz says. The fear, he says, whether valid or not, is that the Centers for Medicare and Medicaid Services may pay less, not more. While he agrees the fear is realistic, he points to the lesson learned when Pap testing became automated. “People complained they didn’t want to move to computer-assisted screening. It was disruptive for them; they had to buy expensive technology. They initially didn’t think it was that great. Yes, it caught all the squamous lesions, but what about that rare endometrial cancer? It wasn’t trained to catch that.” Once there was a CPT code, he says, “almost everyone bought it.”
Hologic’s Quick points to Hologic’s track record. “What we did was work proactively with laboratory customers to develop both clinical and economic data” on outcomes, “not just from the perspective of a lab but from that of a payer.” Are downstream costs avoided? Is patient care better and worth a higher reimbursement? “You need to have that data to change the narrative around CPT coding and pricing.” The CMS is asking for guidance on AI, Quick says, “which is encouraging. But ultimately it needs to go beyond the efficiency of the laboratory. It needs to have a clinical benefit,” and the onus to provide the data, he says, is on industry, clinical laboratories, and hospitals.
A crossroad for many will be whether to believe the AI, Dr. Pantanowitz says. “If you look at a routine prostate case and there’s a heat map over a few glands, and the algorithm is saying, ‘These glands are adenocarcinoma,’ and you do not think it’s adenocarcinoma, you have a predicament.” He participated in a validation study of the Ibex Medical Analytics Galen platform for prostate core needle biopsies at the University of Pittsburgh Medical Center when he was on faculty there. In that study, he says, “we compared AI to MDs,” and there were 30 slides over which such discrepancies arose. They resolved the disagreements through consensus review with colleagues and experts. Ancillary studies also may be done if applicable, he notes. “And I suspect it would be the same in clinical practice.” In their study, he says, the AI was correct in all 30 cases. (Dr. Pantanowitz serves on Ibex’s medical advisory board. Galen received FDA breakthrough device designation in June 2021.)
In future practice, he says, “you won’t have to fight the computer machine—it’s not you versus the terminator. You can ask for help from your partners, and you can run other stains.” If manufacturers set it up so the AI makes a recommendation but the pathologist can weigh in and overrule it, “that seems reasonable,” he says.
Dr. Pantanowitz and his coauthors write in the review article that “AI-based algorithms may seem much more capable than they really are.” Humans are unable to fully comprehend, they write, how “millions of parameters contribute to a decision, leading to potential biases, misuse, and misdiagnoses.”

Dr. Retamero
Dr. Retamero tells CAP TODAY, “We may be limited in understanding how the computer reaches certain conclusions, but part of the pathologist’s role in the diagnostic process when aided by AI is to question what the AI is telling you.” If what the AI says elicits a strong negative response, he says, “that probably means the AI is not accurate,” and that one’s own judgment may be more accurate. “All diagnostic tests produce false-negatives and false-positives, and AI is no exception here. The pathologist has the final say in the diagnosis. In that regard it’s no different than any immunohistochemistry assay or genetic assay,” he adds.
Hologic, Quick says, is beginning to use the term “digital assay”—which the company uses already to refer to its molecular testing offerings—to describe the content that will run on the Genius platform. They’re viewing the system “not just as a replacement for the ThinPrep imaging system, which is a natural progression, but as the creation of a platform for future technologies.” The company’s road map involves building out the menu of digital assays that will run on the Genius platform, including content looking at, among other things, endometrial and ovarian cancer.
“Exciting but incredibly complex” is how Quick describes AI in health care today, noting the regulatory environment is challenging and an opportunity for partnerships between industry and others. “We’ve built algorithms in the past and they’re locked down and we don’t touch them for years. That’s going to change in the future, but it will require a different regulatory strategy,” he says.
Dr. Pantanowitz, with his eye, too, on the future, says AI will change pathology practice, “but the way it will change practice will differ for pathologists in different settings.” GU subspecialists at a large academic medical center, for example, often are inundated with large volumes of prostate biopsies. AI-based tools may make their diagnostic work more efficient, allowing more time for research. Generalists working in a community setting, on the other hand, don’t need help with large volumes of biopsies. “What they need help with is diagnostic accuracy when they have a difficult case,” or assistance with second reads and quality control. “But I think pathologists in either setting would welcome AI because it’s beneficial from that point of view.”
And though some have speculated AI may deter new residents from entering pathology, he believes it’s an enticement. “I do think people coming into the field will see AI as more attractive than a 100-year-old microscope.”
Charna Albert is CAP TODAY associate contributing editor.