May 2020—Artificial intelligence tools are enabling for pathologists, not a threat, says Thomas Fuchs, Dr. Sc., director of the computational pathology laboratory at Memorial Sloan Kettering Cancer Center and founder and chief scientific officer of Paige.AI. He and others spoke with CAP TODAY publisher Bob McGonnagle in March about the hype and reality of AI and the tension around it.
“Some pathologists are concerned that autonomous AI will take over their jobs,” says another panelist, Michael Becich, MD, PhD, chairman and distinguished university professor, Department of Biomedical Informatics, University of Pittsburgh School of Medicine. Hence a “human in the loop” approach based on Friedman’s Fundamental Theorem, which he explains as “a physician assisted by a computer will always outperform a computer alone.”
That and more was the focus of the March AI roundtable, which we share here. Others on the panel were Ajit Singh, PhD, Jason Hipp, MD, PhD, Lisa-Jean Clifford, and Esther Abels, MSc.
Dr. Singh, you gave a wonderful presentation on AI at the Executive War College in 2018, which we reported on in CAP TODAY. Help us separate the hype from the reality of AI. There are a lot of people who would like a discussion around that differential, if it can be made. How do you in your mind, and when people talk to you, separate the hype from the reality around AI in 2020?
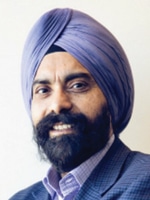
Dr. Singh
Ajit Singh, PhD, managing director and general partner, Artiman Ventures, and adjunct professor, Stanford University Medical Center: Any new technology goes through life cycles. That’s normal. It’s normal for humanity to get excited about something and then realize where the shortcomings are. AI has gone through four hype cycles: in the ’60s, in the ’80s, in the late ’90s, and now. As most of us would appreciate, the basic science of AI has not changed much in the past 50 years. So the original principles set out in the 1970s are pretty intact today in almost the same form.
What has changed is the availability of a tremendous amount of data to learn from, which has been the Achilles heel of AI for many decades, and more computational power. And the computational power was always there; it was just expensive. Now the power is much less expensive. But the key enabling factor is that a tremendous amount of data is available to learn from, and that data keeps increasing.
There’s much more reality now than hype compared with 2018. What has changed? Number one, there’s a clear realization that tackling problems of low dimensionality is going to be a better path to success than tackling problems of high dimensionality. Some of the early failures in AI applied to pathology were that we picked the problems of the highest number of variables in the beginning, thinking, perhaps subconsciously, that if we can solve the most complex issues first, the easier ones should be easy. But it turned out to be not true.
And the reason is not so much the inherent scientific difficulty of high-dimensionality problems but that the higher the number of dimensions, the more data you need. And in many cases that data didn’t exist. So that’s one reality that has seeped in and hence our propensity now to tackle problems of lower dimensionality.
Number two is that we’re tackling use cases that make sense. If you pick a use case—I want to beat the expert, or I want to beat the expert most of the time, or I want to beat the expert more consistently, because at times even the expert might make an error because of fatigue or other reasons—that is going to be problematic, because of the mathematical complexity as well as resistance to adoption. Versus use cases picked because I would rather bring the knowledge of experts to nonexperts, from academic medical centers to community practice, from places that have large amounts of data to communities that have no data at all. That’s a much more viable use case.
Dr. Hipp, let me ask you to react to Dr. Singh’s comments or just answer this question about hype versus reality—how you help make this differential for your colleagues who are curious about this.
Jason Hipp, MD, PhD, senior director and head of pathology data science and innovation, translational medicine, AstraZeneca: I’m very supportive of what Ajit said, and the component I would emphasize for pathologists is that the rate limiting step is often access to these datasets, whether it’s labeled outcome or having pathologists annotate these images. There’s a great need for and a shortage of pathologists doing annotations, which are the labeling of individual cells or tumor types or tumor cells. It’s important to have clean data, in addition to just getting large-scale information, where we know more specifically the outcomes. We all want to do these experiments but don’t have the data access to do them.
Lisa-Jean Clifford, what’s your reaction to what you’ve heard so far?

Clifford
Lisa-Jean Clifford, chief operating officer and chief strategy officer, Gestalt Diagnostics, Spokane, Wash.: It gets to the crux of the issue, and it all goes back to the data. Having access to both the images and large datasets that include the annotations and metadata that go along with the images, as Jason said, is critical to being able to train machine learning and to being able to get to the different dimensions, as Ajit said.
But the bigger issue then becomes the outcome, as Jason pointed out. In many instances, we don’t have the follow-up information to close the loop on the patient. To provide the most robust and detailed analysis, you need the final diagnosis and outcomes for the cases tied back to the images. This includes any historical visits and diagnosis tied to each case. What we are trying to get to is a faster diagnosis and the treatments to best impact outcomes. The core of the information is the data, and being able to capture, train, and disseminate that information.
Dr. Fuchs, what would you say is the proper distinction in 2020 between the hype and reality of AI for pathology and laboratory medicine?
Thomas Fuchs, Dr. Sc., founder and chief scientific officer of Paige.AI; director of computational pathology laboratory, Memorial Sloan Kettering Cancer Center; and professor of machine learning, Weill Cornell: That’s an intrinsic question to our discussion and warrants hours of treatment. On a very high level, AI or machine learning is here to stay because it’s just the function of the data—clinical data and of course images. And that data won’t go away. The names might change—we might use AI or not AI—but large-scale statistical learning, as is done in machine learning, is here to stay because the data is going to grow drastically and will allow for even more powerful models over time.
Nevertheless, in these gold rush times there’s an enormous amount of hype, which is dangerous for the field because models or systems that are not tested in a proper way can lead to disillusionment among practitioners and clinicians. The negative impact can touch everyone in the field. That’s why it’s important that regulatory agencies take a close look at what they approve, to keep the standard high.
We are in a time when we should move forward, and the COVID-19 crisis shows the importance of digital approaches and how they can help in practice with efficiency or even allowing community pathologists or doctors to be as good as subspecialist experts. I’m optimistic about all of that, but all of us, as part of this community, have to be careful in how we advertise it and remind everyone every day that one good number in an ROC curve is not enough to show the performance of a system.
Dr. Becich, what are pathologists asking you regarding this hype-reality distinction? And please talk about SpIntellx. Give us an explanation of your concept of “explainable” AI. I’m still looking at a paper you gave me about a year ago and enjoying that concept. So tell our readers what that’s all about.
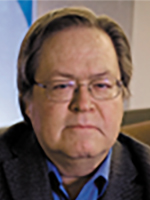
Dr. Becich
Michael Becich, MD, PhD, chairman and distinguished university professor, Department of Biomedical Informatics, and professor of pathology, information sciences, telecommunication, and clinical/translational sciences, University of Pittsburgh School of Medicine: I’m here wearing two hats, one as an academic leader in biomedical informatics and computational pathology at Pitt, and second as a founder of an AI startup, SpIntellx. For maximizing adoption, practicing pathologists need to understand what algorithms are in terms of assistance in making diagnostic calls in whole slide images, which is the focus of one of two products at SpIntellx.
We’re working with dozens of pathologists across the nation at academic centers and in private practice as well as commercial laboratories, each of which we think approaches this from a different perspective. We want to put explainable AI and machine learning, artificial intelligence tools, in the hands of the diagnostics decision-makers. At SpIntellx our approach is really “human in the loop.” We run AI algorithms as part of the surgical pathology workflow, integrated with the LIS and focused on improving the efficiency of whole slide image interpretation, and empower—and not replace—pathologists. This will enable significant opportunities to use AI in arenas where pathologist shortages exist and expertise is not available.
Explainable AI is important for the following reason: Some pathologists are concerned that autonomous AI will take over their jobs. That remains a problem, even with whole slide imaging and implementing it into practice. Our approach is based on something called Friedman’s Fundamental Theorem, which says that a human alone will never outperform a human using a computer enhanced by artificial intelligence. In that theorem, explainable AI gives the power back to pathologists for the ultimate decision but gives them checks and balances, quality control, and feedback from what the AI algorithms call, to inform accurate decision-making. The approach we’re taking is providing this feedback linking human intelligence to computer intelligence in the framework of pop-up windows that come along with the derived value our algorithms give to help guide the pathologist efficiently toward regions of interest critical in making the diagnosis.
One of our first products at SpIntellx will help increase the speed of whole slide imaging reads by pathologists with augmented intelligence that guides them to key regions of interest. This intelligent aid will result in a 50 percent faster read on whole slide images and get them comfortable with using AI in practice today.
It’s important to get AI into high-volume commercial practices and to private practice pathologists, where 98 percent of diagnostic pathology occurs first, and then to back propagate it into the tougher settings in academic health systems, which will be the early adopters, and we’ll be doing this at UPMC as well.
Esther Abels, it’s clear that AI and digital pathology are coupled. They have some of the same problems not only of regulatory and financial justification but also technological development.
But at the root of it they have historically, and still today, created fear about job displacement in pathology. Virtually everyone on this call, including you, is also deeply experienced in digital pathology. Can you give us a sense of where this unease of the profession may be headed and how the AI cycle relates to the digital pathology cycle?
Esther Abels, MSc, vice president of regulatory affairs, clinical affairs, and strategic business development, PathAI, Boston: I fully agree with what Mike said, that the pathologist is always there and that AI will guide the pathologist in informed decision-making. We can never replace a human with AI. It will be so with self-driving cars, in aerospace, everywhere. And I’m referring here to ethics and the mind. We are ultimately trained to have our core values, and based on that we make decisions, and that’s something that AI will not be able to do. We can train them as such, but we cannot give them the mind of a human being.
Second, fully automated algorithms are coming, just as we see in genomics. In genomic sequencing, a report will be generated, and then an outcome, or result, is there, and based on that, the physician, the health care provider, can act. We’re heading that way as well with regard to digital pathology and AI. But we all know there are limitations in training algorithms and we must be transparent on what those limitations are. How did we train the algorithm? What was the input data? What were the limitations? From a regulatory perspective, especially in the U.S. and highly likely also in Europe with the In Vitro Diagnostic Regulations becoming effective soon, the questions will be: What initially is true, is it still true, and can we transfer this to the population from individual models? The regulatory authorities will regulate to the population, not on the individual level. As long as we’re transparent in what it is, how we train it, then we can guide the pathologist in making those better decisions and helping them become more confident in using digital pathology, including algorithms. You will have more of the life cycle of pathology itself, whole slide imaging systems plus multiple algorithms that can be run in parallel. There will be more adoption, and there will likely be a big shift in payment.
Lisa-Jean, considering the almost epic experience we’ve had, particularly in the United States, with regulation around digital pathology, how do we envision that AI will go any better? Or are we in for yet another difficult regulatory confrontation?
Lisa-Jean Clifford (Gestalt): The issue is that we haven’t resolved the regulatory limitations—not really requirements, but limitations as they apply to digital today. To support its ability to be used widespread, we need to get past that hurdle first. Then AI will follow, but I believe it needs to be a fundamental education around the value of AI and digital pathology being used in practice. If we are able to get the regulatory bodies over the challenge of understanding what the benefits are of digital pathology and artificial intelligence, then I think we can remove the perception of mystique associated with them.
There should not still be any concern that a pathologist can diagnose from home where they have no tissue samples, no instrumentation, no reagents, and where what they are using at home is what they are using today in their office at the lab: a computer and monitors. An office that meets HIPAA requirements and has followed the self-validation process should be approved for use regardless of its location.
Dr. Hipp, do you have a comment about the profession’s anxiety and the worry about regulatory roadblocks, in this one sense of the word, along with the coupling of digital pathology and AI?
Dr. Hipp (AstraZeneca): As a pathologist who’s also doing AI research, here’s how I see it, especially in the near-term: AI’s going to be a tool for pathologists, just like IHC is a tool for pathologists. People thought originally IHC could detect tumors, meaning we don’t need pathologists now; everyone will just run a brown stain and tell us what it is.
This will empower us in two ways with pathology. It will help us make diagnoses, and, from the drug development perspective, potentially help in designing new companion diagnostics that can identify features within tumor cells that pathologists might not identify or might not be able to calculate every cell shape and size of the nuclei and nucleoli. So we can use this as a tool to reinvestigate the H&E and bring the H&E back to life. And it can help pathologists with tedious tasks, such as counting tumor-infiltrating lymphocytes, and understanding what’s going on with the tumor biology when we’re giving patients therapeutics, for new drugs especially.
Pathologists need to be involved in conducting this research because this is our discipline. We’ve been answering these questions with rudimentary tools. Now it’s an opportunity to come back with these powerful quantitative and consistent tools.
Dr. Singh, let me hear your reactions to these comments.
Dr. Singh (Artiman): I’d like to pick up on two connected issues and on the issue of the “kit” that Jason brought up. And Mike brought up the issue of explainability, which is critical and ties to the regulatory issue Esther raised. The notion of explainability goes back to the early days of AI, in 1956 when the Dartmouth conference took place with four tracks. And one of the tracks that [John] McCarthy [one of AI’s founders] himself led was on causality. And this topic was then further picked up by Judea Pearl in the ’90s. He wrote a beautiful book in 2018 that I had a chance to review [The Book of Why: The New Science of Cause and Effect, published by Basic Books].
I’m going to read one brief paragraph from there, and that’ll tie us back to this issue. He says, “As I reread Genesis for the 100th time, I noticed a nuance that had somehow eluded my attention for all those years. When God asks Adam, hiding in the garden, he asks, ‘Have you eaten from the tree from which I forbade you?’ And Adam answers, ‘The woman you gave me for a companion, she gave me fruit from the tree, and hence I ate.’ ‘What have you done?’ God asks Eve. She replies, ‘The serpent deceived me, and hence I ate.’”
The question was a “what” question; the answer was a “why” answer. Humans are wired to answer the question why, and unless the why is understood, we don’t believe it. It goes back to the issue of explainability. If we can explain how we reached an outcome to a diagnosis or a conclusion, or a confusion for that matter, it is then palatable to everyone. It’s palatability to the people in the profession, namely pathologists, that will be critical to get their buy-in. It is even more critical to get the buy-in of the regulatory bodies.
Regulatory bodies historically have had a position that they will not approve a black box. It has to be a white box or at least a gray box. And that constraint is not an illogical constraint and hence we must tackle the issue of explainability. Which means for the time being, putting a human in the loop will be critical. But there are companies and institutions that are building the notion of causality into AI systems, especially as they apply to pathology.
Often causality is not tied to a single modality. How do we get causality as humans? We connect the dots from other things we have learned, which is a multimodal way of reasoning, and we get confirmation of our intuitive hypotheses. Similarly pathology is then reinforced to other modalities, be it next-gen sequencing data, data coming from clinical history, or data coming from prior such references. That will have to go into causal explanation, which means it’s not just digital pathology. It would have to work in conjunction with others. Ultimately, this will lead to robustness that will be relevant for our discussions with the regulatory bodies.
It’s a tool, Jason, you’re right. In my days with BioImagene, roughly 12 years ago—this was before Roche acquired BioImagene—we had used the phrase, “The algorithm is the kit.” Much the way Sun Microsystems said in the 1980s “the network is the computer.” The algorithm is the kit, and the more we give it the semblance of a kit, the more we make it feel like a kit, the more it will be used as a tool and accepted as one.
Dr. Fuchs, you’re right in the middle of this as a founder and chief scientific officer. What is your reaction to what you’re hearing around these notions of explicable AI and the effect on the profession of pathology?
Dr. Fuchs (MSK): Jason mentioned something that’s very important: These tools and assays we are building are enabling for pathologists. It’s not only for efficiency, and it’s not only for the pathologist, but it’s also for all of the oncologists. There’s a lot of work in predicting all kinds of information outcome, response, et cetera from H&E images, and that puts the pathologist much more in the center of the patient treatment process, as the main diagnostician. In my experience at least, the vast majority of pathologists see that future and embrace it. It’s not age dependent. Young and experienced pathologists are very much looking forward to the future where they can reason about more interesting things than counting Ki-67 and so forth. So this mortal threat is not there, especially because it is the extension of previous work in the field.
These models are not black boxes. They’re completely transparent. You can investigate every weight, every connection, every design choice. They are complicated boxes, but they are not black boxes. In contrast to a human brain, you can investigate biases, you can correct for biases, you can do all kinds of tests and validation of these algorithms. You cannot do that with human experts.
Regarding explainability, we have been through that many times in machine learning. Just looking back at random forecasts, when they came out nobody wanted to use them because they’re so complicated compared with logistic regression. And why would anybody do something non-linear if you can do a logistic regression? And there were many groups that worked on visualization, explainability, and today it’s an off-the-shelf tool. The regulatory bodies pause not even for a second if you come up with that.
We’re seeing the same thing in deep learning. There are many groups, specifically those in pathology, that focus on explainability, and on the commercial side there’s not a single company that would advertise the replacement of pathologists. So these are tools, and if the tools are used the right way, they’re enormously empowering for pathologists, especially for pathologists in community practices, in large labs, and in the rest of the world. If you look beyond the ivory towers we have the luxury to live in, these tools will help patients in countries with health care disparities, in areas where the resources are not as plentiful as at Memorial or at UPMC.
Dr. Hipp, companies like Genentech and AstraZeneca are still reporting that there’s resistance to understanding this new role of the biomarker as driving therapy and of the pathologist as being the ultimate diagnostician. We sometimes call this the “town versus gown disparity.” Can you comment on the disparity and attitudes among pathologists, particularly in the community, as being perhaps not quite so eager to fulfill the new role that Dr. Fuchs so properly outlined, which is the new role for pathologists in our world?
Dr. Hipp (AstraZeneca): I don’t see it from the AstraZeneca side as much as being in the field of digital pathology for the past nine years, going to residents’ forums, conferences, and other events.
How we communicate these messages is what’s important. You have to speak multiple languages when you talk to this audience. You have to speak to a pathologist in the context of being a pathologist and understanding what it’s like and what you go through and the pressures you’re under. But you also have to use these quantitative terminologies—for example, explaining complex engineering where few pathologists have an engineering background.
C. P. Snow talked about the differences between humanities and science and speaking to the different worlds and languages. It comes down to communication and how I’ve been viewing and explaining things. Pathologists would always come up to me and say, “Oh, you’re trying to put me out of a job.” And I would explain, “No. This is going to make us better.” Then I would feel like I got a lot of buy-in and support and understanding.
That’s how we can communicate these messages and make more pathologists advocates for this technology—speaking to them as pathologists but yet understanding the science. More pathology informatics fellowships are crucial for the field as we begin to address this and to serve as the bridge between two different disciplines that never talked to each other before. And I’d love to hear the group’s thoughts on that.
Let’s start with Dr. Becich.
Dr. Becich (UPMC): This is a complex set of issues, but in pathology it should start with our member organizations, and, unfortunately, pathology’s member organizations are about as fragmented as they’ve ever been. The largest with a lobbying arm and the most financially successful is the CAP, and the CAP has begun to give a voice to what we need to do to engage community pathologists.
How we train our pathologists is where we’re not doing our job. To me, those are two significant choke points for infusing AI into pathology practice. The third choke point is with our LIS vendors, largely asleep at the wheel today in informing how we, in real time, start to infuse these technologies into pathology practice. Until those three choke points are alleviated, we’ll be a brute-force academic and industry innovation partnership to change it by disrupting this discipline.
Because of genomics, whole slide imaging, and the rise of microbiomics, particularly in the era now with infectious disease affecting us all, we can no longer stand behind the stovepipe industry of partners we have. And we have to transform our leadership in pathology practice from understanding how the community is informed by what’s happening at academic centers like Memorial and Pittsburgh and Michigan and Ohio State and other places that are now trying hard to push this gigantic ball up the hill to transform the culture of pathology practice.
Lisa-Jean, what’s your reaction?
Lisa-Jean Clifford (Gestalt): I agree with the need to be able to speak the correct language. I hear a similar story from many of the pathologists I work with in terms of embracing technology now and into the future. And age doesn’t matter—we are seeing pathologists of all ages and specialties becoming engaged with, and embracing, digital pathology.
What I am hearing are concerns over the use of AI. Some of the concern, and I see this more from pathologists at regional laboratories, is that AI will replace them. But what I’m hearing more often relates to the black box comment that was made. I agree that those of us who understand the technology know it is not a black box and that algorithms are fully transparent and well documented. The comment that resonated with me was how we have to speak the correct language to pathologists so that they understand and are comfortable with the fact that algorithms are locked once they are validated. I have had more than one pathologist state that they thought algorithms would continue to grow, evolve, and learn on their own indefinitely. The concern was that the algorithm used this minute isn’t going to be the same as the one used in 10 minutes, for example. It all goes back to speaking that correct language to the pathologist.
Our knowledge as the developers of the technology and of how it is used in practice needs to be communicated to the pathologists and the adopting laboratories in a language they understand and are comfortable with.
Esther, what’s your reaction to what you’ve heard? And the challenges of acceptance and alleviation of untoward anxiety?

Abels
Esther Abels (PathAI): It started when I was with Phillips when we got the imaging device cleared by FDA. We thought true clinical adoption was going to happen as soon as the first device, which was already being used in the clinical setting in Europe, would get to the market in the U.S. Then we got the clearance from the FDA, but still, the true clinical adoption at scale was slower than we all anticipated. Now looking at that, and when talking with users, pathologists, and vendors, for me what sticks out as a challenge comes back to interoperability.
In Europe, adoption is a little ahead of the curve compared with what’s happening in the U.S. We can learn from them. We can also learn from aerospace and automotive because there has been a lot of adoption and artificial intelligence usage already. Europe is ahead because its interoperability is better than in the U.S. I agree the LIS vendors could step up, but I also think the government can step up and make interoperability a regulation of sorts. I’m not in favor of adding regulations to health care, but I am in favor of guiding it or regulating it so that it becomes easier. We have to overcome all these hurdles to move this technology forward.
Dr. Singh, one of the concepts you talked about in your 2018 presentation at the Executive War College was the importance for innovation of what you call “cognitive diversity” or “transdisciplinarity.” Do you believe that pathology as you know it or experienced it has adequate transdisciplinarity?
Dr. Singh (Artiman): We can certainly learn from nature. If we appreciate the diversity in the Galapagos Islands and reason for a bit where it comes from, it’s obvious the importance of biodiversity there and how it leads to nature’s platform of innovation, which is life. Our human-made innovation is no different. It also requires diversity, among other parameters, and in this case it’s cognitive diversity. Jason made the point of how few full-fledged engineers have taken on pathology as a profession. That kind of answers the question.
There is a hierarchy of transdisciplinarity and I want to call out a couple such levels in this hierarchy. One is the transdisciplinarity across fields from engineering, AI, pathology per se, cell biology. There’s a transdisciplinarity at that level. Then there’s a transdisciplinarity at the integrative level because at the end we should not conceive the role as a pathologist only. Rather, we should conceive the role of a diagnostician, and as a diagnostician there has to be integration from multiple modalities of diagnosis, diagnostic tools, if you will. That’s the second or higher tier of transdisciplinarity that we need to incorporate. At Stanford we have a program on integrated diagnostics, and this is center stage in that subject.
On a scale of zero through 10, if I could summarize my comment, we are probably at a two or three in terms of transdisciplinarity.
Dr. Becich, where would you put us on a scale of one to 10 on the richness of our transdisciplinarity?
Dr. Becich (UPMC): Let me start with pathology in general. If 10 is perfect, pathologists are about a 0.9 in terms of their transdisciplinarity. That’s historical because pathologists generally get disrespected in medicine, often aren’t at the board tables when financial decisions are made, and aren’t necessarily the world’s best communicators. From the standpoint of academic pathology, most communications that go outbound to medicine do come from academic centers.
The largely discohesive nature of member organizations, as well as our member-facing organizations in pathology, leave us too fragmented to have a major impact and voice. Look at what’s happening with COVID right now. Pathology should be in the front and center in terms of understanding the importance of getting testing out there efficiently.
In academic health centers, diversity comes from who we put in our pipeline. We have a robust STEM pipeline to bring diverse and female voices to an issue that’s largely male dominated in technology and STEM. We need to retrain people in pathology. We need to embrace our communities, and if folks want to learn more about our approaches at Pittsburgh, I’m happy to talk about them.
Dr. Hipp, a final comment from you?

Dr. Hipp
Dr. Hipp (AstraZeneca): I’d like to come back to this transdisciplinary concept in pathology. I’ve found it easier in industry to do multidisciplinary research, especially as a pathologist because I’m not on service. I don’t have to worry about grants. Often what I noticed is that in our health system we had the pathologists in the basement with their microscopes and the Center for
Engineering in a different part of the graduate school. So there’s that proximity divide. Whether I was in Silicon Valley or in the pharmaceutical industry, you sit in proximity. I sit next to the oncologists, the engineers, the bioinformaticians, and you need to have that kind of community. That idea where you don’t have to send an e-mail and wait for it to come back. You have that real-time discussion, that brainstorming.
Dr. Fuchs (MSK): I agree that interdisciplinary understanding and language and the willingness to get involved is more crucial than ever. But that’s true for all other disciplines these days so pathology is not unique in that sense. And in my experience, the vast majority of pathologists are eager to be part of the future and go forward boldly. There are always detractors and the detractors sometimes are loud. But we should not fall into false equivalency where we think that the fear of replacing pathologists is as big as the enthusiasm of the vast majority of pathologists who move forward to a better future and make the discipline much better.
Someone touched on education and that’s key. In Europe as well as in the U.S., pathologists must make pathology cool again. It’s important to present it as a modern and central discipline, which it is. I’m positive about the future.
Dr. Singh, how would you like to sum up your experience on the panel, your final thoughts, and if you might, what advice would you give for CAP TODAY readers in light of our roundtable?
Dr. Singh (Artiman): As always, anything that is philosophical, strategic, in discussion must end with something practical. So, at the end, what do we do? What’s the practical implementation? There are three pieces of general guidance.
Number one, digitize, digitize, digitize. Even if we have no idea how we’re going to use it, just digitize. Collect whatever data we can collect in our profession. If the incremental cost or effort of collecting one extra field of data is minimal, collect it, even if we have no idea today how it’s going to be used four, five, 10 years from now.
Number two, index, index, index. Whatever we can do in terms of creating some sort of a meta structure on top of data, do it. Even if you don’t have a program today in AI in a certain institution, just do whatever indexing is possible.
Third, annotate, annotate, annotate. Metadata is critical, and that means physical annotations by experts today, namely pathologists, is critical.
Even if we have no program in place, even if we have no idea how we’re going to use it, just making the data available in a digital form for your use and potentially others’ use in a syndicated, federated manner is going to push the field much further along than we can imagine.