Machine-learning algorithms: how to detect and deter bias
November 2022—Bias—a type of prejudice that may go back to the beginning of humankind—has, in recent years, been the focus of attention with regard to developing machine-learning algorithms for clinical laboratory testing.
Clinical laboratory tests can hide data biases that could potentially generate misleading or even inaccurate results, says Brian Jackson, MD, medical director of business development, information technology, and support services, ARUP Laboratories, and associate professor of pathology, University of Utah School of Medicine, Salt Lake City. “When an algorithm gives you an answer to a question, but the algorithm doesn’t display the logic by which it arrives at that answer, the user is left at a big disadvantage in being able to see whether there’s bias going on.”
To compensate for such potential issues, laboratories need to focus heavily on quality control before implementing tests based on machine-learning algorithms, Dr. Jackson says. “I think the validation needs to be even more aggressive than what we do for [other] laboratory tests,” he adds.
Common types of biases that affect machine-learning algorithms include sample bias, exclusion bias, and measurement bias, says Christopher McCudden, PhD, clinical biochemist, Ottawa Hospital, and vice chair, Department of Pathology and Laboratory Medicine, University of Ottawa, Ontario. Dr. McCudden is also deputy chief medical/scientific officer for the Eastern Ontario Regional Laboratory Association.
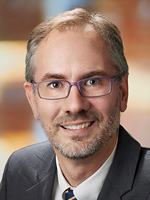
Dr. Jackson
In cases of sample bias, he says, the data used to create an algorithm are not fundamentally applicable to the population for which they are being used—for example, using data from male patients to create an algorithm for testing women. In exclusion bias, a portion of a population is omitted from the data, effectively creating a data set that doesn’t represent the population as a whole. Measurement bias occurs when an algorithm is applied to a population using equipment or a test method that differs from what was used to create the algorithm.
All of these types of biases have the potential to generate flawed laboratory results that can negatively affect patient care, Dr. McCudden says. Complicating matters further, sample and exclusion biases can be based on many factors, including race, gender, diet, and age.
Biases, Dr. Jackson adds, can be found in all algorithms that are based on heterogeneous data sets because smaller subgroups within a population are, by definition, underrepresented in results. “The machine-learning algorithm is going to be mathematically reflective of the 95 percent, not the five percent,” he explains. “So, basically, the biases that exist in real-world data sets end up being represented in machine-learning algorithms in ways that are completely invisible to the user.”
For laboratories, the first critical step in mitigating machine-learning bias is being able to access the data sets used to develop the tests, Dr. Jackson says. Ideally, researchers should have access to the original data on which algorithms were tested so they can determine whether they can replicate the results.
A University of Pittsburgh School of Medicine study comparing various algorithms for predicting the mortality risk for pneumonia patients illustrated the importance of transparency, Dr. Jackson says. One of the algorithmic models that the researchers studied “learned” a surprising rule suggesting that pneumonia patients with asthma had a lower risk of developing more severe pneumonia. (An independent analysis of the study was published in KDD ’15: Proceedings of the 21st ACM SIGKDD International Conference on Knowledge Discovery and Data Mining [Caruana R, et al. doi.org/ 10.1145/2783258.2788613].)
A closer look at the data revealed why that was the case: “It reflected a true pattern in the training data—patients with a history of asthma who presented with pneumonia were admitted not only to the hospital but directly to the ICU,” according to the article. In other words, pneumonia patients with asthma were being treated more aggressively, which could have contributed to their lower mortality risk.
A challenge for the study researchers was that while neural network algorithms, or neural nets, were the most accurate algorithms for predicting high-risk pneumonia patients, they were not transparent. The flawed asthma rule was discovered using a less accurate but more transparent logical-regression algorithm. Consequently, “although the neural nets were the most accurate models, after careful consideration they were considered too risky for use on real patients and logical regression was used instead,” the article authors explained.
In peer-reviewed studies of algorithms, it is essential to examine the methods sections for details about test specificity, sensitivity, and predictive value, as well as how the algorithm was evaluated, Dr. McCudden says. “How big a test population was used? Who were the people? How accurate was it? Is it generalizable? You want to beat up the algorithm to see whether it would work in your particular population.”

Dr. McCudden
But Dr. McCudden acknowledges that peer-reviewed studies may not contain all the information about an algorithm’s methodology that is necessary to achieve transparency. Algorithm developers sometimes claim that privacy concerns prevent them from disclosing all data about study participants. In addition, the methods sections of peer-reviewed articles often have limited space, so technical information about how data were generated, such as which instruments were used with which specimens, may not be included.
Commercially-developed machine-learning algorithms are even more opaque, Dr. McCudden says. Often the best option, he adds, is to treat the commercial algorithm like an unknown or unproven lab test and conduct proficiency testing with specimens that have already been diagnosed.
“If you know that people have disease X and this algorithm is supposed to detect it, does it? If you know that people do not have the disease, does the algorithm indicate that they don’t have it? Calculate the performance metrics and see whether it meets expectations,” he advises.
Laboratories looking for help in mitigating bias in machine-learning algorithms can find expert resources online, Dr. McCudden says. Google’s responsible AI practices website, for example, outlines the company’s recommended best practices for avoiding artificial intelligence bias. IBM’s AI Fairness 360 is an open-source toolkit of metrics that can be used to check for bias in data sets and machine-learning models, and it provides algorithms for mitigating such biases. In addition, the Alan Turing Institute’s fairness, transparency, and privacy interest group publishes research and other information online that can help identify and mitigate machine-learning bias.
Yet, regulators, including the FDA, have been slow to adopt rules and protocols for addressing machine-learning bias in laboratory tests, Dr. Jackson says. The FDA has held conferences and workshops on the topic, but “it appears that the FDA had been underregulating this area, and I hope they step up,” he adds.
Dr. Jackson chairs the ethics subgroup of the CAP Artificial Intelligence Committee, which is developing a position statement about ethical responsibility related to the use of AI in pathology and lab medicine. The AI committee hopes to submit the statement to CAP leadership for consideration by the end of the year.
“Any health care organization looking into implementing a machine-learning algorithm has a responsibility to ensure that appropriate validation has been done,” Dr. Jackson concludes.
—Renee Caruthers
Clinisys completes integration of companies under its brand
Clinisys has completed the process of combining Sunquest Information Systems, Horizon Lab Systems, and ApolloLIMS under its brand.
“We are consolidating behind the Clinisys brand to signal our expanded support for a much wider range of industries and sectors, as well as global operations,” said Clinisys CEO Michael Simpson, in a company press statement. “As we look to the future, we will continue to invest in our core products and solutions from each of the combined businesses as part of our long-term integrated Clinisys Laboratory platform strategy.”
Clinisys, 520-570-2000
Gestalt Diagnostics and mTuitive collaborate
Gestalt Diagnostics and mTuitive have entered a strategic partnership to interface Gestalt’s PathFlow solution with mTuitive’s CAP eFRM electronic forms and reporting module, developed in partnership with the College of American Pathologists.
PathFlow is a cloud-based digital pathology platform that is made up of professional, educational, and research modules. CAP eFRM captures diagnostic data in the CAP cancer protocols via the electronic cancer checklists to support CAP and American College of Surgeons accreditation requirements.
“The newly created interface between the two will create a seamless workflow that utilizes the key data captured in both mTuitive solutions as well as Gestalt Diagnostics’ solutions,” according to a Gestalt press release.
Gestalt Diagnostics, 509-492-4912
NovoPath offers new version of lab system
NovoPath has added capabilities to its NovoPath 360 cloud-based laboratory information systems via its winter 2022 release.
Users of NovoPath 360 can now add ancillary molecular tests to any surgical workflow linked to a patient case and produce one report for all tests. Furthermore, the system provides automatic continuous reporting.
Additional features available with the winter release include:
- out-of-the-box preconfigured workflows.
- role-based permissions that control access to the LIS based on a user’s authorization level.
- customization features that allow laboratory administrators to add, edit, rename, reorder, and delete fields within the application and configure reports or views to reflect the lab’s workflow.
NovoPath, 732-329-3209
GoMeyra adds test panels to LIMS
The cloud-based software-as-a-service company GoMeyra has expanded its GoMeyra laboratory information management system to encompass monkeypox, urinary tract infections, and sexually-transmitted infections.
The customizable LIMS uses digital scanning to process test samples. It includes functionality for sample storage, testing, reporting, and archiving.
GoMeyra, 702-846-3962
Proscia updates digital pathology platform
Proscia has introduced a new version of its Concentriq Dx digital pathology platform for primary diagnostic workflows.
The latest release of Concentriq Dx supports peer reviews, conferencing, consults, and tumor boards. It enhances live collaboration among remote teams by allowing participants to make annotations, communicate via chat capability, and take control of the viewer via fellow mode.
The platform also contains an enterprise administration module that puts control of roles and permissions, as well as site-specific settings, in the hands of lab administrators.
Concentriq Dx is CE-marked under the European In Vitro Diagnostic Medical Devices Regulation. It is available for primary diagnosis in the United States during the COVID-19 pandemic under an FDA emergency use authorization.
Proscia, 215-608-5411
Indica Labs and Hamamatsu extend relationship
Indica Labs and Hamamatsu Photonics K.K. have announced an agreement to maintain long-term interoperability between Indica’s Halo AP anatomic pathology software and Hamamatsu’s NanoZoomer family of high-speed, high-resolution scanners.
“This agreement is an important reassurance to our mutual customers that both companies are committed to working together on technical, regulatory, and commercial fronts,” said Indica Labs CEO Steven Hashagen, in a company press release.
HALO AP is CE-IVD marked for use in primary diagnosis in the European Economic Area, Switzerland, and the United Kingdom. It is available for research use only in the United States.
Indica Labs, 505-492-0979
Labgnostic contracts with TriCore and Arkana labs
Labgnostic has contracted for its Labgnostic data- and systems-agnostic connectivity hub with TriCore clinical laboratory, Albuquerque, NM, and Arkana Laboratories, Little Rock, Ark.
Laboratories linked to the Labgnostic hub can send diagnostic test referrals and results to others participating in the network via a single interface and track specimens exchanged with participating laboratories.
Labgnostic, 424-367-8081
Dr. Aller practices clinical informatics in Southern California. He can be reached at raller@usc.edu. Dennis Winsten is founder of Dennis Winsten & Associates, Healthcare Systems Consultants. He can be reached at dwinsten.az@gmail.com.