November 2023—Generative artificial intelligence—what it is, how it can be used in pathology, what stands in its way, why the excitement. CAP TODAY publisher Bob McGonnagle spoke about that and more with pathologists Bobbi Pritt, MD, MSc, and Scott Anderson, MD; Ajit Singh, PhD, of Stanford and Artiman Ventures; and Devon Snedden, a health care consultant in artificial intelligence.
“There are a lot of excellent possibilities that we’re just starting to understand and explore for the field of pathology,” said Dr. Pritt of Mayo Clinic.
Here is what she and others said when they met online Sept. 12.
Ajit Singh, why has the excitement for generative artificial intelligence picked up in the past two years?
Ajit Singh, PhD, managing director and general partner, Artiman Ventures, and adjunct professor, Stanford University Medical Center: Interest in AI as applied to health care has been around forever, and within health care it’s been in the areas of diagnostics and therapy selection and, more recently, drug discovery and clinical trials. In the early days of AI in health care, in 1968, the first applications were in the selection of the right antibiotics. So what changed in recent decades? First, the amount of data in the public domain exploded thanks to the likes of Google and interoperability. Second, computational power became inexpensive. Everything that was not possible in the self-learning systems became possible because of these two reasons.
A few things are still not possible. While there are enough data, there are not enough data with corner cases, and in medicine it’s about the corner cases. Generative AI helps us solve that problem via data augmentation. It helps create additional data from which to learn. It helps predict what kind of data there could be lurking around the proverbial corners. If there is such a thing as a holy grail in AI, it is not that AI works; it’s that AI works in the corner cases.
Scott Anderson, what questions are uppermost in your mind about generative AI?
Scott Anderson, MD, professor and director, cytopathology, Department of Pathology and Laboratory Medicine, University of Vermont Medical Center: How can we leverage generative AI to help pathology education at the graduate medical education level, clinician-interaction level, and undergraduate medical education level? There’s been a shift to moving toward active learning and figuring out how to engage students in a way that they learn on their own, with pathologists or other experts there to help them understand the process. Generative AI can help make that process more efficient for pathologists, who could use it to build cases and provide explanations for students and other learners.
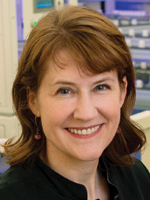
Dr. Pritt
Bobbi Pritt, what do you want readers of CAP TODAY to know as they think about generative AI?
Bobbi Pritt, MD, MSc, professor of laboratory medicine and pathology and interim chair, Department of Laboratory Medicine and Pathology, Mayo Clinic, Rochester, Minn.: We have publicly available data sets; we can use privately available, more curated data sets; and we have the computing power. How do we harness that to be useful for pathologists? I’m a CAP governor and a member of the CAP Information Technology Leadership Committee, and within the committee we have a project team looking at how generative AI can help pathologists and laboratorians in the United States and around the world.
As the name implies, generative AI generates new data. Even though we tend to think of things like ChatGPT as answering questions, it could also generate data for data sets. It could help us generate images, perhaps even histology images. It has broad implications across education but also for compiling reports that patients and patient-facing clinicians can understand, and providing ways for pathologists to do their job better. I want readers to know that the CAP is actively looking into this.
Ajit Singh, generative AI is the cherry on top of the AI sundae in that it seems to be creative. Something is being created that wasn’t there before and it’s on top of a great deal of genuine data. Is that where some of this excitement is coming from?
Dr. Singh (Artiman): As far as the excitement and hype is concerned, yes. But let’s step back and say what it really is. Generative AI is statistically predicting the next data point. Let’s use language as an example. It’s saying that given everything everyone has said that’s in the public domain, what is statistically most likely the word or phoneme or phrase that will be said next. In that sense, is it really creative? If I use the philosophical definition of creative, no; it’s not creative at all. Now the question is, can we harness the power of being able to predict statistically what is likely to be next? In a one-dimensional version it applies to language. In two- and three-dimensional versions it applies to images. And maybe in four- and five-dimensional versions it applies to other complex data such as oceanography, weather, climate, potentially earthquakes.
Coming back to medicine, if we have the ability to generate data that doesn’t exist yet, what could it be used for? It could be used for training. It could be used for augmenting data to create a larger data set to learn from. Most importantly, it can be used to generate corner cases, the unlikely edge and corner events where even the best pathologists will get stuck and need a second or third opinion. It probably has the highest utility there.
I’m going to translate this data speak into history. Every so often a new manuscript of a scrap of music by Mozart or Beethoven or Chopin is found and it arouses curiosity among the experts of those composers, including the performers and people who have studied them all their lives. This is a kind of corner case that Ajit describes. We now have a piece of music by Mozart, but we don’t know when it was written. We have to figure out what stage of his evolution is accounted for by this manuscript, how it might be played, and how it might sound, which are not trivial problems to musicologists and performers. Devon Snedden, generative AI would have a role to play for musicologists here, would it not?
Devon Snedden, MGM, health care consultant in artificial intelligence: Generative AI empowers us to sequence together bits of information that already exist into a new opera, maybe. Right now we know what constitutes real-world data. Many guidances define what good data looks like and there are clinicians who can interpret that data. The dilemma for those clinicians is that they don’t get the full picture. Generative AI allows us to sequence together real-world data, to some extent even observational data, and provide real-world evidence. It allows us to make real-time—not retrospective—treatment decisions and look at the entirety of a patient, whether that’s image based or assay based or all of it. It not only creates a new clinical picture for the patient but also a comprehensive clinical picture that’s not otherwise able to exist.
For instance, if Dr. Pritt or Dr. Anderson wanted to do a deep dive on one of their patients, they could try to collect all the patient data that includes all records, and after days, weeks, or months they would probably be able to make a different evaluation—one that’s more comprehensive for the patient. Generative AI empowers the clinician to put those things together and operate in real time.
Dr. Singh (Artiman): In music or any work of art you have to have gone through the pain and suffering to produce good art; there is no shortcut to that. In medicine there are so many unknown parameters but you have to make decisions despite them. You have to have gone through the bottom-up learning process that develops over a dozen years and suddenly all the synapses are firing in synchrony, so the opera does happen there.
Where is the application? Let’s assume the diagnosis is somewhat understood and the specifics at the molecular level of the diagnosis are available, and now I have to select therapy. If I choose just standards of care, let’s say for non-small cell lung carcinoma, there are probably 70 regimens between first, second, and third line, and often it’s the third-line regimen that’s going to work. Isn’t there a way to bring it to first line? If it’s for refractory patients or end of life patients and the physician wants to use something off-label, there are even more choices. For therapy selection there are two ways to get to it. One is, I want this outcome; what options do I have? It’s an inductive technique. I want to try—in concept, not actual practice—1 million options, 100,000 options, 10,000 options, or 70 options, as in the case of non-small cell lung cancer. Which one is likely to produce the outcome I want? Generative AI allows me to try all these paths in parallel to generate what is likely to happen progressively across all 70 options and then pick the one that generates the outcome I want. Hence, when somebody does inductive inference of what is the likely treatment that will produce the best outcome for this patient, generative AI will have a tremendous role in therapy selection.
Bobbi Pritt, what’s your reaction to that?
Dr. Pritt (Mayo Clinic): There are a lot of excellent possibilities that we’re just starting to understand and explore for the field of pathology. There are ethical and accuracy concerns about generative AI, and we have to keep those in mind. Human expertise should remain the final authority in pathological diagnosis.
Dr. Singh (Artiman): I agree. We will never replace the physician. Any statement that says a physician can be replaced by AI—it’s nonsense.
There are roughly 4 billion people in the world who do not have access to a primary physician, let alone an oncologist. What is their alternative today? Do nothing. Can AI do far better than do nothing, which is like tossing a coin? Yes. If I have 80 percent positive predictive value of a diagnosis with an AI system, should I lament about the PPV being 20 percent less than perfect? My response is no. Rather, it’s 30 percent better than tossing a coin. So the context matters. If I’m in Houston or New York, no issue. If I’m in Tuscaloosa, Alabama, or Sioux City, Iowa, I have a slightly bigger problem. And if I’m in Malawi, the problem is to an extreme. That context determines to which degree we deploy AI either as an adjunct to a physician or as an alternative to a physician.
Devon Snedden (consultant): When I was in laboratory technology school in the late ’90s, instrumentation was reaching a different pitch of application than it had in previous decades, and I remember my mentors in the laboratory saying we were all going to be replaced by instrumentation and be out of a job. It turned out we were able to process things much better in the laboratory and it empowered the laboratorian and pathologist to do their jobs better. I see generative AI, with the appropriate checks and balances and risk evaluation, being deployed in a similar manner.
Dr. Pritt (Mayo Clinic): Yes, generative AI and other forms of artificial intelligence are tools that will allow pathologists and laboratorians to do our jobs better. It won’t replace us, but if we don’t embrace these technologies and use them as they become clinically validated, then we may be replaced by pathologists and laboratorians who do use them effectively. It’s important for pathologists and laboratorians to follow this field closely and understand how these tools can help them but not replace them.
Dr. Anderson (UVM Medical Center): It’s important for institutions that train pathologists to look at this and embrace it to help the next generation of pathologists work. They’re going to do it regardless, with or without us. It’s important for us to help guide them in that process.
If you think about the democratization that generative AI makes possible—Ajit refers to places in the world where the coin toss is standard of care—it’s mind-boggling. Do you agree?
Dr. Anderson (UVM Medical Center): Yes. There are a lot of quality training programs that have varying levels of content. Utilizing AI may enable the sharing of content. Generative AI may help equalize different programs and training across the country.
Devon Snedden, if I’m at the Texas Medical Center maybe I don’t need another large hotel to house the people from all over the world who travel to get their care onsite. Perhaps we have better alternatives for them. Mayo Clinic, Cleveland Clinic, and others are bringing the institution overseas and not having people fly in. Do you detect some of that in your work?
Devon Snedden (consultant): Absolutely. This is a top-of-mind conversation for how we are going to democratize care and provide access not just to academicians but to practicing clinicians anywhere. You don’t necessarily need to have the data scientist onsite interpreting and building the access points. The insights that generative AI affords can be generated at an institutional level or outsourced to an alliance or registry, for example, and be provided where they don’t otherwise exist or are inaccessible. Not only does it allow care to be brought to places where it otherwise wouldn’t be available, it also allows the data to be aggregated in ways it wasn’t before. We’re building these solutions now for clinical oncologists, in radiology, and downstream in different ways.
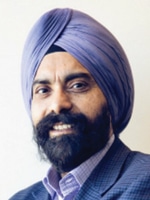
Dr. Singh
Dr. Singh (Artiman): To illustrate Devon’s point: In 2008 there was an earthquake in Haiti. I had just started as the CEO of BioImagene and we were about to deploy an instrument to a paying customer in Los Angeles. We said let’s not deploy it there; let’s ship to Haiti. One of our interns took it upon herself to take one of our scanners and set up a tent in a camp in Haiti, and we set up a digital pathology link in Miami. We screened 3,000 patient microbiology slides a day. We were able to provide a first opinion.
Let’s assume another scenario where the first opinion is available locally and we provide a second opinion, better than what would have been available locally. The second opinion could also have biases. Let’s assume we had third, fourth, fifth, sixth, and seventh opinions coming from generative AI systems that have been trained on different data sets. If the generative AI is trained on the same data set, it will have similar biases. But if five systems are trained on five different data sets, they are orthogonal to each other. Using them simultaneously to provide first, second, third, fourth, or fifth opinions—or let’s create a new term for it, simultaneous multi-opinion—we will have the ultimate proof of democratization. We shouldn’t eliminate the human from the loop. We should help humans eliminate their bias.
Devon Snedden (consultant): Functional biases exist also because of poor clinical data interoperability, and the solutions now are manual and never without risk. We can use generative AI to support clinical data interoperability from a top-down perspective and empower laboratories to provide better data. When you look at the applications in practice management and how you’re going to empower practices to evaluate their care and decide what to insource and outsource, you can help payers realize the effectiveness of a diagnostic or a therapeutic that would otherwise take them years of diagnostic observational data to be convinced of the same evidence.
What may be standing in the way? We know there are vested interests that stand in the way of anything that’s progressive. We also know in other fields there are people who rush in to invest, which leads to too many players and then the need to rationalize the companies and investment in the field. Ajit Singh, where are we with that in generative AI?
Dr. Singh (Artiman): I am seeing generative AI applications more in pharma than in diagnostics. There are potentially two reasons. First, it’s the lower-hanging fruit. Pharma generally pays well. The decision cycles are quicker than in diagnostics, partly because you can go with a research-use-only product and it can be a path to regulatory approval. Second, there’s probably a genuine, useful application in the early stages of the drug discovery cycle when you’re generating hypotheses, and the ability to generate a lot more hypotheses and pick out the more likely ones up front could eliminate problems downstream. For every company I see in the diagnostic field, I see 15 to 20 in pharma applications. It bodes well for diagnostics because tools developed for life sciences often end up in clinical routine diagnostics sooner or later.
Bobbi Pritt, what obstacles do you see?
Dr. Pritt (Mayo Clinic): One potential obstacle is getting your laboratorians and pathologists to embrace new technologies such as AI. In the Department of Laboratory Medicine and Pathology at Mayo, we try to involve the people who are the end users of new technology in the evaluation of new systems and in their subsequent validation and implementation. Get the people who are boots on the ground using the system, get their buy-in, and let them drive the processes rather than have it come from higher up. We’ve found this to greatly improve the acceptance and use of AI in our workflows.
For example, we just implemented a deep learning neural network in our parasitology laboratory. It’s been locked down for clinical use and has shown excellent accuracy and sensitivity for detecting protozoan parasites in trichrome-stained stool specimens. Our technical specialists and technologists initially evaluated two commercially available AI algorithms and ultimately chose the system they thought worked best. I oversaw the process and defined the evaluation study but let the lab drive the process. Our technologists then performed the validation of the system and drove its integration into our laboratory workflows. Now they love it and are our strongest advocates for its use. Things could have gone much differently if I were the one who had dictated the selection and use of the AI system and told the technologists it was going to change the way they worked.
Devon Snedden, when I think of funding and getting through barriers to new technology, I think of the federal government—the NCI, NIH. What do you see?
Devon Snedden (consultant): Federal agencies have been able to harness the implications of the 21st Century Cures Act. The Cures Act says you have to leverage real-world evidence and help provide patients with access to their data. I see those components reinforced most.
The Center for Biologics Evaluation and Research and the Center for Drug Evaluation and Research recently released guidance on real-world evidence that will operationalize how we are going to implement some generative AI applications. From a federal health perspective, many of the agencies understand that the data must be aggregated for clinicians and clinician practitioners, and they’re looking at the best ways to aggregate and provide access to that data.
The biggest obstacle I see is in the more functional components. Not long ago we were still having the conversation about digital pathology and how to implement it in satellite locations. That conversation shouldn’t have persisted because we all understood that digital pathology had utility. We face a similar analog in this environment, which is how do we provide access and not leave it up to the health care system to decide what the boundaries of risk are. I see a lot of guidance coming out around what exists and should exist for real-world evidence and data and how those should be generated.
Ajit Singh, what are your thoughts about the immediate obstacles to data aggregation as they apply now in health care to generative AI?
Dr. Singh (Artiman): I’m reminded of radiology modalities that went digital in the late ’80s, when PACS [picture archiving and communication system] first came about and obtaining native digital data was possible. For the first five years of PACS, nobody worried about storage, interoperability, and aggregation across multiple sites, as long as each radiologist had their own workstation and there was connectivity to the referring clinician. Next came the need to have large amounts of storage to aggregate across a facility, and soon we realized that problem was more complicated. Hospitals were buying out other systems, and the problem was almost insurmountable, even in technical terms—sheer, raw compute power, storage power, interconnectivity. The problem was further complicated because of different standards and ontologies. This is the least interesting problem intellectually in the domain of data aggregation but nevertheless very important to address.
Fast-forward to now. The amount of data is about five to six orders of magnitude more. We often confuse big data with a lot of data. Big data implies too many free variables in the data and not just “a lot of data.” Even a small amount of data with many variables has a lot more value. The complexity is greater as well.
The rate-limiting factors are, to some degree today, storage and compute power. But having a lack of common ontologies is a much bigger problem.
Devon, where are we with ontology?
Devon Snedden (consultant): I’ll counteroffer a term: semantic interoperability. Semantic interoperability must include many different data sets. CPT codes, RxNorm, SNOMED, FHIR [Fast Healthcare Interoperability Resources]—all of these must be integrated. But if we’re looking at creating something that’s immediately accessible for physicians and patients, we also have to take into account the extraordinary amount of data that already exists and is usable. I see generative AI as able to not only consume all of those in ways that would be out of the question manually but also create a data picture that is interoperable and immediately applicable. As Ajit said, it is the least interesting challenge in the room but the most prohibitive in creating a full clinical picture.
Dr. Singh (Artiman): Generative AI can play a role in generating ontologies. It may be left to humans, then, to select and aggregate the right ones. But ontology creation is difficult and the number of variables is large—generative AI can play a role there. It will be counterintuitive because essentially what generative AI does is each time they have new data, they make the model even bigger. The task of abstraction is a reductionist task. We have to make the model smaller, and that’s the role of ontology.
Bobbi Pritt, with the devotion to pathology informatics at Mayo, are your colleagues focusing on these issues in the way we’re discussing today?
Dr. Pritt (Mayo Clinic): Yes. Mayo Clinic has the institutional Center of Digital Health, and our Department of Laboratory Medicine and Pathology has a new division called CPAI—computational pathology and artificial intelligence. CPAI is taking a department-wide look at how computational pathology and AI can be implemented across all divisions—for example, microbiology, transfusion medicine, hematopathology, and anatomic pathology—and determining their impact to our medical practice. They are also creating standardized processes for evaluating, validating, and implementing these technologies. For example, they have created an AI life cycle and guidelines for validating new artificial intelligence algorithms, just like you would validate any other type of new laboratory test. Our pathologists and laboratory directors aren’t currently very comfortable validating artificial intelligence algorithms, so CPAI will play a key role in helping our divisions adopt new technologies in a thoughtful way.
Scott Anderson, what’s the reaction you’re getting from the residents and prospective residents you talk to? Do these topics excite them, bore them, or are they focused on becoming a subspecialty surgical pathologist or hematopathologist?
Dr. Anderson (UVM Medical Center): I’m not sure that AI is top of mind quite yet. It has exploded in the last couple of years, so I think it will become top of mind relatively quickly and we’ll see more and more people who have been trained using AI or are at least exposed to it in medical school or using it independently to increase their knowledge.
There’s certainly a push around digital pathology for whole slide imaging; that’s always brought up by prospective residents, and I see that as an extension of AI. It’s going to take time before it becomes top of mind for everybody, but we will see a push from the newer generation.
Devon Snedden, is there something you would like to mention that we haven’t touched on?
Devon Snedden (consultant): Is there an opportunity to use generative AI to generate a more comprehensive report for a treating clinician? We’ve discussed educational materials for the patient. Is there a way, using generative AI, to transform clinical information for a patient in a language that is more comprehensible?
Scott Anderson, is there anything you’d like to raise?
Dr. Anderson (UVM Medical Center): I’d add to what Devon said about patient educational materials and the value that generative AI could have in helping not only patients but also clinicians who are curious about pathology. Providing consultative services through that would be interesting and have a lot of utility.

Dr. Anderson
Ajit Singh?
Dr. Singh (Artiman): How well do these systems perform? Whenever a new collection of technologies is brought to a new application, we expect perfection. When HIPAA technologies were coming together, our expectation was that they had to be perfect. Every field has to be auditable, et cetera. The existing state at that time was that I could wear a white coat, put a stethoscope around my neck, walk into any file room at my hospital, and pick any file I wanted. But with an information system, we expected perfection.
Let’s step back and think of the use cases where generative AI is good enough. Will it be perfect? The goalpost will keep going back, so it will never be perfect. But there are many applications today for which the performance is good enough, and it behooves us to start the application pilots in those areas.
Bobbi Pritt, do you have a comment to conclude today’s conversation?
Dr. Pritt (Mayo Clinic): This was an inspiring and informative conversation, and the CAP and the project team that Dr. Anderson and I are leading will be looking for ways that generative AI can help pathologists and at what the concerns and hurdles may be. We will continue to disseminate that information to CAP members, so stay tuned for more.